Machine Learning Techniques to Optimize Inventory and Improve Service Levels
Companies are all looking to keep inventory costs as low as possible while keeping customer service at its highest level. More recently, resilience of the supply chain has taken the front seat to cost and profit! To this end, zero-inventory and just-in-time concepts of past decades have lost their validity. The use of AI and machine learning techniques has opened many new solutions to tackle what is known as one of the oldest problems in the world: How much do I need?
There are four main areas of supply chain planning to focus on when trying to get more from your inventory investment. From top to bottom, and with different time-horizons, each one is critical to get the whole picture right, so it’s important to target them individually:
The Four Areas of Supply Chain Inventory Planners Should Focus On
1) Reduce forecast error with better Demand Planning using machine learning
2) Establish better inventory target levels with prescriptive algorithms such as Multi-Echelon Inventory Optimization (MEIO)
3) Synchronize supply flow with S&OP and S&OE and predict (learn) supplier customer, and operations patterns using machine learning
4) Use machine learning algorithms to improve safety stock values and predict disruptions to reduce risk and improve resilience
Reducing Forecast Error
The two key factors that will impact the amount of inventory that is required in a supply chain are supplier lead-times and demand uncertainty. Although, inventory planning and management forecasts are not always perfect, there is a great deal that can be done to increase its accuracy with improvements in process and technology. Forecasts and “consensus demand” (i.e. aggregation and agreement on one forecast number, by all departments) are also used to determine forecast error. So, if a company does not have a strong process in place to facilitate collaboration, they will not be able to do well in any of the other areas. Forecasting algorithms are a great starting point especially with the use of machine learning techniques, however processes must be put in place to decide the design of forecasting policies for old and new products and who the stakeholders are. In addition, systems can monitor accuracy of contribution by different participants and adjust the weight of their input accordingly.
Multi Echelon Inventory Optimization (MEIO)
Where and how much to place inventory can be very difficult to figure out in an end-to-end supply chain with many products, regions and customers. There are many ways to rebalance how inventory budgets are allocated. Plus, inventory pooling and production postponement strategies can be complex and hard to execute, as planned. A Multi-Echelon Inventory Optimization (MEIO) system will enable a company to consider all of these in deciding where in the supply chain and how much inventory to have at each stage of the supply chain. If your company is using a manual system, (thus, pretty much guessing at how many days of coverage to have for each product) or does not have a good process in place to calculate statistical safety stock values and its “What-if” impact on customer service, then you should be looking into how an MEIO system can help your supply chain. MEIO, is basically an AI technique using gradient descent search, that provides a trade-off between cost and service level for different customers and products. It also defines the most optimal levels of inventory based on your service level and cost trade off.
Sales and Operations Planning
As part of the S&OP and S&OE process a company needs to determine how to meet the inventory demand that comes from buffer stocks, forecasted demand, and backlog. Or it may be that capacity or material constraints, or other operating efficiency concerns, drive a company to purchase or build inventory ahead of when it’s actually needed. Changes in throughput or reliability of manufacturing process, and the amount of WIP, all have implications in what inventory is needed and when. Regardless, the supply planning process that feeds a consensus S&OP plan is the first place that these decisions are made. Except that, S&OP solutions do not consider the effects of finite capacity, materials, and operating constraints that were mentioned earlier. To this end, control over inventory levels will not be achieved effectively unless we have an integrated S&OE solution to respond to short term disruptions from suppliers, WIP changes, manufacturing issues, or sudden changes in demand, both up or down. In a planning continuum, S&OP and S&OE are done within the same system using a true digital supply chain twin. All that is needed is granularity and frequency of data for the system.
Safety Stock Calculations Using Machine Learning
Safety stock requirements tend to change depending on many factors such as demand changes, seasonal variations, product life cycle, regional events, availability of resources, and expected weather disruptions for certain regions, just to name a few. Machine learning algorithms help to find the underlying patterns in the supply chain that impacts the need for safety stock. Based on these patterns and their root causes, they can predict what the right level of safety stock should be for each product (family), customer or a region. Such inventory planning and management techniques are highly reliable and can have a drastic impact on inventory costs and service levels.
In summary, there are many areas of supply chain inventory planning and management that can have a significant impact on reducing inventory and improving customer service. Typically, a company will focus on demand planning first, and then inventory management, while putting in place simple ways to set inventory target levels such as replenishment planning. They would then focus on better inventory targets with MEIO systems and better supply side strategies such as supply planning, safety stock calculations and more predictable execution using S&OE solutions. For a complete guide to supply chain strategies and use of AI/ML in supply chain planning click here.
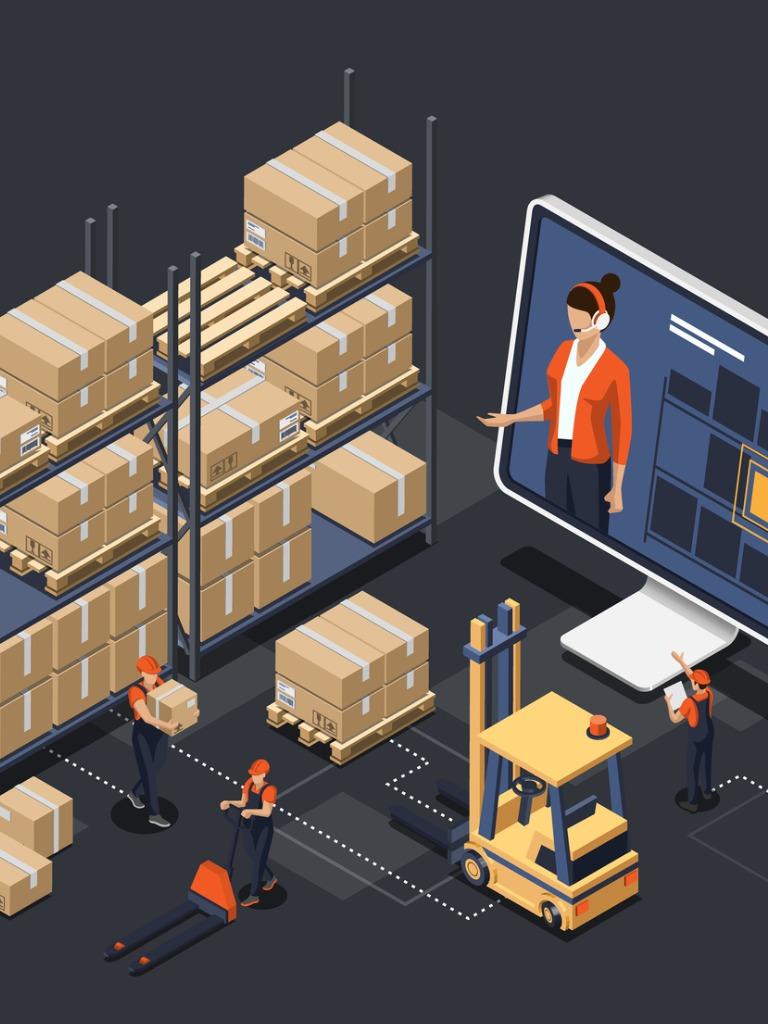