Generative AI and Supply Chain Planning
In a recent survey by Gartner more than one third of the respondents indicated that improving decision making robustness, speed and quality are their top three goals. Furthermore, 85% of business leaders have experienced decision stress, and three-quarters have seen the daily volume of decisions they need to make increase tenfold over the last three years. When it comes to supply chains, decision making is key to reducing costs, delivering on time and increasing market share. In a large company, typically there are tens of planners trying to plan, respond to events and maximize use of resources as best as they can. There are also tens of others who are trying to execute the plans that have been passed on to them. How good were the plans in the first place remains to be unknown. At best these plans were made either manually using spreadsheets, or use of scenario analysis or an optimization system that assumes everything is predictable with no uncertainty is built into the plan. It merely provides visibility and leaves it to humans to solve the problem. Humans cannot make decision fast enough, robust enough and with high quality in real-time. The result is a decision that will just be ok, not knowing how good or optimal this decision is.
Given the fact that supply chains are becoming more complex and growing, and the pressure to take into account not just cost and profit but also ESG factors, many leaders have turned to AI for help and Gen AI to seek answers. Certain AI techniques such as machine learning have been around for many years and are currently used in demand planning and far less in supply planning. In contrast to demand planning, supply planning is treated by most systems as deterministic. The best use of AI comes from predicting uncertainty and using it to enhance quality of decisions. To this end, stochastic planning assumes an environment where supply characteristics are changing all the time depending on many factors such as seasonality, supplier performance, geo-political and socio-economic factors. Using this information, it creates plans that are superior. For example, considering oil price variation can improve the quality of plan and produce better mix of products increasing profits. Other examples are changes in efficiency of resources and supplier lead-time variability can lead to better on-time delivery and lower inventory.
Gen AI or Large Language Models (LLM) are very good if there are enough examples for them to learn from. They can learn very fast and act as if they are experts. They can learn text and be able to interpret messages, write code, diagnose medical issues or tell stories and write movie scripts. However, they are not good at and cannot perform numerical analysis and calculation. This requires a vast number of examples for them to learn from either algorithms or experts. But the experts, as mentioned earlier, do not necessarily know what the best answer is to every event. Even if they did, would they provide enough examples for the system to learn from. There are also limitations to learning from algorithms. Foundation models that are derived from LLMs require a very large amount of diverse data which is not available to them due to privacy and copyright issues.
Gen AI, however, can be used to improve planner productivity but it is not suitable for plan optimization and improvement of operations. It can do much of the routine work of planners such as reading and evaluating emails and messages, it can provide answers to some questions they might have or help them to find the right sources of information and prioritize their daily tasks. However, it cannot improve delivery performance, shorten cycle times, rearrange the mix of products, use the substitute products, respond to a shipping delay and/or a surprise order or improve sourcing percentages while optimizing operations and use of resources. Such tasks are undertaken using several planning and execution systems. Not just S&OP but S&OP combined with S&OE to eliminate data and decision latency and perform stochastic planning for uncertainty. The result is an antifragile supply chain that keeps getting better. For more information on the above concepts click Here.
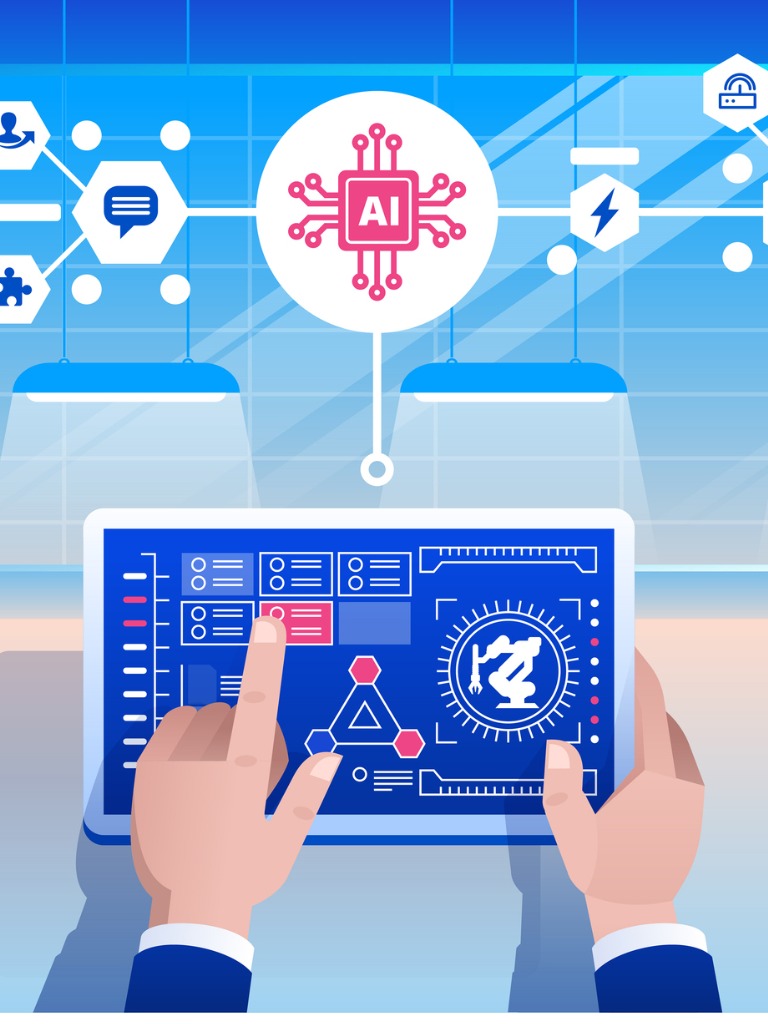